Source:Rounding the Earth Author:Mathew Crawford
After teaching multiple classes yesterday, I napped and then pulled an all-nighter to get out the draft of what will hopefully be my first scientific publication (I was somehow left off the paper in 1995 after I derived the runtime algorithm for the Basic Local Alignment Search Toolkit used to compare evolutionary distances between species. Being a naive seventeen-year-old in science sucks, I guess). This is all likely going to get very interesting, but before I explain what I’ve been working on, I’d like to explain why it is that the data—all the data—from all the hydroxychloroquine studies to date, which looks undeniably positive, should look even more positive than this picture:
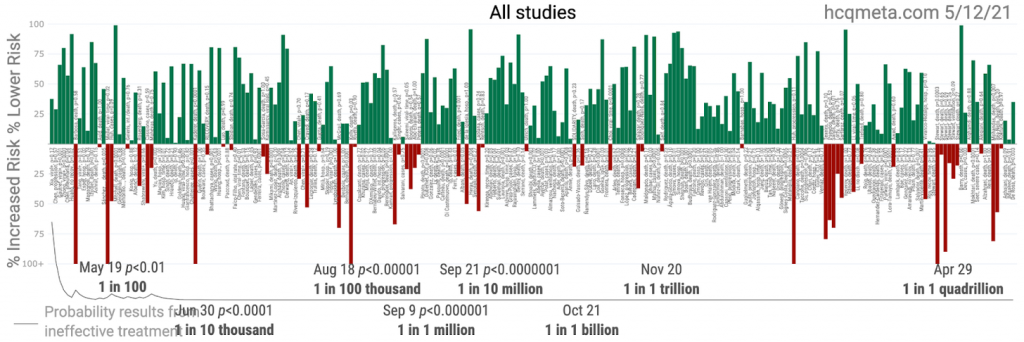
And I’m not talking about the well-discussed flaws of the WHO trials (which used doses higher than any doctors in the world are using or would ever advise, without antibiotics usually coupled with HCQ treatment, and without vitamin/zinc packs associated with the best outcomes, and which were similar in loading dose to the Brazilian trial in which the highly dosed patients started dying rapidly and in great numbers, and mostly on patients who were left to rot until severe COVID-19 already set in). Nor am I talking about the five trials that used reactive agents in place of placebos (here, here, here, here, and here) that might be the only five medical trials anyone I’ve talked to has ever heard of that made such a mistake or possibly a “mistake“.
If you’re thinking this has something to do with “optimal treatment plan” or something similar and that probably seems foreign or “anecdotal” to our hard working public health authorities, you’re not exactly wrong. But it’s still probably not what you’re thinking. Even this picture isolating the early HCQ treatment data should look better than it does, and potentially far better:

In a previous article, I explained the Simpson’s paradox, but I’ll go a little further this time.
Consider the hypothetical effective antiviral drug XYZ. Let us endow this mythical drug with the ability to reduce mortality by 60% when administered to patients immediately upon progression from mild to moderate COVID-19, which is usually the point at which hospitalization occurs. Now, consider three hospitals with three distinct treatment protocols, each of which treats 1,000 patients:

The first hospital medicates patients with XYZ immediately upon presentation. The second hospital has a “compassionate use” policy and lets patients languish for a little while before medicating them with XYZ upon reaching the severe COVID-19 disease state (I know, right? “compassionate”). The third hospital provides “standard of care”, which means isolating patients, serving them hospital food, having nurses prone them or produce a dance video for tik-tok, then maybe hooking those patients up to ventilators if they haven’t gotten up and gone home. Apparently, comparing hospitals 2 and 3, XYZ still has a 20% mortality benefit when administered at the severe COVID-19 onset. Why we would ever amass the data to find that out about an antiviral, I’m still not sure. But a lot of the real world data during the pandemic includes observations like this that never should have been possible. SCIENCE!

Now look at the analysis at the bottom of the chart. The patients who received drug XYZ died more often. And if you’re thinking this is some kind of dark voodoo, I don’t blame you. But it’s really just a Simpson’s paradox. This happens because the XYZ treatment group includes the higher risk patients from Hospital 2, and the non-treatment (control) group gets the benefit of all the patients in Hospital 2 who were healthy enough to recover quickly, powered by their own immune systems (that’s a thing, I hear).
At this point, there is a group of lazy, fearful, unimaginative, or sociopathic biostatisticians who ram the data into some nifty matrix and say the magic words, “Bayesian correction” and get some numbers closer to our hypothetical efficacy assumption, however still imperfect, but so obscured from the original data that nobody could argue with it who doesn’t already understand how the analysis should have been conducted. Some problem solvers might think to group the first two hospitals together, but that also comes up with the wrong answer:

Now we see a 40% reduction in mortality, but that’s still pretty far from 60%. What gives?
The only way to see the full effect of drug XYZ is to treat each hospital (or their protocol, really) as its own cohort. Then we compare hospitals 1 and 3 and see the full 60% effect, as promised.
Without going into great detail (though the proof is not hard for a mathematician and probably plenty of high school students with the talent), given that drug XYZ has an antiviral effect, any data aggregation between different protocols has a monotonic and negative impact on any computed effect size. That could mean dampening the measured effect size, or even a reversal of those measurements, as in the Simpson’s paradox, making the drug appear deadly. And this impacts a whole lot of the studies, both observational and RCTs, that have been published during the pandemic, including a lot of the HCQ efficacy studies.
But there’s another catch. Even this 60% number doesn’t explain the full extent of the problem! Sorry to lead you on, but this part seems to elude a great number of scientists and medical professionals, so I’m going to take great care in explaining it as simply as I possibly can. Here goes…
Nothing in this mock analysis…nothing at all…tells us…regardless of any and all Bayesian voodoo Voldemort can summon…nothing we have seen tells us the most important thing that we need to know…which is…
the level of efficacy of antiviral XYZ, when used optimally, which is to say immediately upon first symptoms at the mild COVID-19 stage.
BAM! Did your brain just snap out of the Fauci trance you didn’t even realize you were in? You didn’t even realize there were multiple grades of pharmaceutical hypnosis that doesn’t involve ingesting colorful pills, did you? Okay. Never let it happen again.

Regardless of any hospital data published, none of it tells us anything at all about the effect of using HCQ, and in particular within the best protocols that might pair it with an antibiotic (azithromycin or doxycycline in particular), zinc, vitamins (C and D and others), and possibly in conjunction with additional options such as ivermectin, albuterol, budesonide, and maybe monoclonal antibodies for a few patients. It could be that early ambulatory multidrug regimens that incorporate HCQ reduce mortality by as much as 100%.
If you’re wondering why nobody ever told any of us that…yeah, I’m still wondering, too. This should not be difficult logic for an analytical mind working professionally with medical data. But it should be understood that most of the people hired to handle that data are not “the best of the best” (Wall Street would have sucked em right up, if not Google or Facebook or some intelligence agency other than Google or Facebook), and they are taught to run through the same formulaic procedures over and over and over again until they’re self-hypnotized by the process. Not all of them. But maybe all of them. Maybe. I do suspect there are a few, quietly navigating the dark forest they didn’t know they were getting into, looking for ways to do good without alarming anyone. But if the pharmaceutical industry ever caught wind of them, they’d likely be slated for early retirement.
So, then, how do we know how good the effects of early, optimal treatment of multidrug regimens might be?
Glad you asked, because that was essentially the project that Dr. Brian Tyson tasked me with analyzing and writing up when he contacted me a few weeks ago. Early during the pandemic, many doctors were well aware of the potential promise HCQ held in treating coronavirus patients. In fact, hospitals were stocking up on HCQ in mid-March, prior to HCQ’s Trump moment (even if the media didn’t tell you so). But while political psychology did its thing, making HCQ a no go zone for many physicians, Tyson and his staff simply treated patients according to whatever seemed to work, using HCQ as a primary option. And work it did. The multidrug regimen used at Tyson’s Valley Urgent Care centers in Imperial County, California (a county which incidentally suffered one of the worst case fatality rates in the nation at over 3%) was applied to thousands of patients with remarkable results. Once Tyson was sure of the reality of those results, he got the word out through some interviews, but that still left Fauci and public health authorities with the most obnoxious excuse not to…encourage the immediate use of medicine…ever employed: “the evidence is ‘anecdotal’ so just sit at home and maybe get sicker until your oxygen drops and you need hospitalization for acute disease while we negotiate the production of a few billion jabs” [and we’re not going to bother collecting the early treatment data so that it remains anecdotal forever and ever and ever].

If you’ve read this far, you have my gratitude, and now you get the prize. While Dr. Tyson knew his results were good, he did not realize just how good they would be when viewed through the lens of time/severity stratification. I spent two weeks cleaning and organizing the data, and some time writing the paper. Here are the preliminary results:
- Out of 3,962 patients presenting with mild symptoms, there were 2 hospitalizations and 0 fatalities (spelled “z-e-r-o f-a-t-a-l-i-t-i-e-s”). These represent 99.76% and 100% reductions relative to Imperial County numbers, after correction for demographics.
- Out of 413 patients presenting with moderate symptoms, there were 7 hospitalizations and 3 fatalities. These numbers represent 94.3% and 67.5% risk reductions by the same metrics.
The latter numbers look a lot like drug XYZ. And the former numbers, where Dr. Tyson and his team did the right thing (the logical thing) and treated patients very early after symptoms began, could not get much better. In fact, you would expect several more hospitalizations from that many people during an average one week period after visiting Valley Urgent Care.
The next step for this research is to pass through the hands of some highly experienced and well published professionals as we shape the paper up for submission to medical journals. And after that? My hope is to get a hold of more early treatment data and perform the same form of analysis, and publish all of it. After enough data for early ambulatory multi-drug regimens, we can fold them into a meta-analysis. While there are early treatment meta-analyses, it is important to isolate what appear to be the most optimal (meaning likely multidrug) treatments and highlight just those. I mean…isn’t policy optimization bent toward saving lives the actual goal?
Author outreach for financial support:
In order to achieve that goal, I need help in casting a kind of protection spell. This is where you come in. Ordinarily, I don’t stop and advertise the paid subscription feature of this newsletter, but this is that moment. If I can step away from my other business activities (I do still teach and write math curriculum and take on consulting gigs), I can focus 50-60 hours a week on the research instead of just the 40 I’ve been putting in for the past three weeks (I’m being straightforward about this). There is no FDA (ha!) or other grant funding this research. Dr. Tyson self-funded the first study simply to get the job done. But I’d like to commit to doing all the research possible and as quickly as possible without having to dip into personal savings. You can help:
- You can forward this article to friends. Make sure these are friends with open minds, aren’t currently engaged in Nazi COVID hall monitor fantasy LARP games while redecorating the living room with framed Fauci portraits, and aren’t hypochondriacs who are going to read this and immediately question the statistical analysis of seventeen medications they’re taking.
- If it’s in the budget, upgrade to a paid subscription. Whether or not you subscribe, most of my articles, and in particular The Chloroquine Wars series, will remain free to view always. Right now maybe one in ten to twenty articles are behind a paywall. So, for the most part, your subscription serves as a donation to an implicit medical grant research pool that ultimately pays my rent and electricity bill. Also, you’ll be buying textbooks for my pupil Antonio, the [very bright] high school dropout who up and decided he could become a professional statistician himself. He is making great progress.
- If you are aware of an organization willing to commit a grant to this project, I’d be sincerely grateful. But the idea of taking a week off myself to thumb through and apply for grants for a highly politicized topic makes me cringe and twitch.
Finally, I’d like to thank all of the readers brave enough to venture here. We live in interesting times. Keep your good humor whenever and wherever possible, and find the ways you can to prepare for harder times that may come. Finding a better world on the other side will take considerable effort, so we might as well make the best of it in the meantime.
Journal of Medicine Says HCQ + Zinc Reduces COVID Deaths
The Chloroquine Wars Part V – A Closer Look at RCTs Studying Hydroxychloroquine Efficacy
The Chloroquine Wars Part VI – The Simple Logic of the Hydroxychloroquine Hypothesis
The Chloroquine Wars Part VIII – Hydroxychloroquine’s Safety Profile and a Cost-Benefit Analysis
The Chloroquine Wars Part X – A Discussion of the Insanity of the Chloroquine Wars
The Chloroquine Wars Part XI – See No Good, Hear No Good, Speak No Good
The Chloroquine Wars Part XII – Manufactured Fear During Hydroxychloroquine’s Trump Moment
The Chloroquine Wars Part XIII -A Clockwork Orange Man
The Chloroquine Wars Part XIV – How to Rig Research: Surgisphere Part I
The Chloroquine Wars Part XVII – Why the Story About India’s April COVID-19 Spike is All Wrong
The Chloroquine Wars Part XV – How to Rig Research: Surgisphere Part II
The Chloroquine Wars Part XIX – Historical Failures of Public Health Authorities