Source: Open Letter
Concerns regarding the misinterpretation of statistical hypothesis testing in clinical trials for COVID-19
This letter is an expression of concern that a significant part of the medical community, and specifically some articles in important medical journals, are misinterpreting the statistical results in randomized clinical trials conducted so far to answer the question regarding the effectiveness of hydroxychloroquine in the early treatment of COVID-19.
Although there is evidence that hydroxychloroquine is not effective in severe hospitalized patients,(1) its use in the early stages of the disease is still under debate.
Recently, three important medical journals have published influential papers about the early use of hydroxychloroquine to COVID-19(2),(3),(4).
Their design limitations aside, they are randomized clinical trials, which are the gold standard in medical research. These three papers have had a substantial impact in the media, on public policies and within the scientific community.
These three papers nevertheless share at least one common mistake: the conclusions they draw from their data are wrong. All three papers lead, explicitly(2),(4) or implicitly(3), to the conclusion that early treatment of COVID-19 patients with hydroxychloroquine is not effective. In saying that the conclusions are wrong we are not affirming that hydroxychloroquine is effective. This is a subtle but important distinction.(5)
The null hypothesis in these articles is defined as H0: treatment effect = control effect. In any classical statisticaltest, the null hypothesis can never be accepted, it can only be not rejected. This is a well known issue.(6)
Randomized trials are widely used in medical science. All these three studies applied a statistical hypothesis test to analyze their results and draw their conclusions. They had similar results: all treatment effects measured in the studies showed positive results, with treatment groups displaying better outcomes than control groups in each variable measured but with non-statistically significant differences at 95%(2),(4) or 90%(3) confidence levels.
The formal conclusion for these hypothesis tests should be that there is not enough evidence, for the sample and test adopted, to reject the null hypothesis that treatment effect size equals control effect size for the chosen confidence level. A more appropriate interpretation of the formal conclusion in these studies would be that there is evidence that treatment effect is positive but this evidence is statistically inconclusive in the sense that it is not possible to conclude, at 95%(2),(4) (90%)(3) confidence level, that the effect could not be attributed to randomness.
In other words, their results bring evidence that early treatment is effective. The confusion happens because evidence is measured by statistical effects, not by p-values, which measure the uncertainty of this evidence. (5)
Large p-values are related to increased uncertainty in the evidence obtained. They can be large for two reasons: one, the treatment is not really effective and the evidence found were due to randomness; two, the sample size was not big enough to measure an actual treatment effect precisely.
Hence, initially at least, if the p-value is not small enough it is not possible to attribute this fact to the treatment effect, since the treatment can be effective and the large p-value could be attributed to a small sample size, a limitation of the study not of the treatment.
Recently, Nature published an editorial to bring attention to the fact that COVID-19 trials sample sizes were too small.(7)
That all three hydroxychloroquine (HC) studies showed positive but inconclusive results suggests they might be underpowered. For example, the largest study aimed at a prior relative effect of 50% to define its sample size. (2)
Although this may not be high when compared to treatments for some other diseases, this seems very ambitious in the COVID-19 context, as shown by the dexamethasone relative effect of 10.8% displayed in table 1 below.
The primary intention of this letter, however, is to call attention to the misinterpretation of the hypothesis test results, not to perform a full analysis of their statistical powers. Therefore, we choose to show in table 1 a plain comparison of a part of their results with those of the celebrated Recovery randomized trial on dexamethasone (DX) for COVID-19.8
Note that the p-values displayed below for hypothetically larger samples are not formal estimates. The intention of the following comparison is mostly to emphasize that p-values cannot be directly compared without taking into consideration the effect sizes they are measuring and the sample sizes used. (9)
We use the dexamethasone paper as a benchmark because the medical and scientific communities largely agree with its importance for COVID-19.
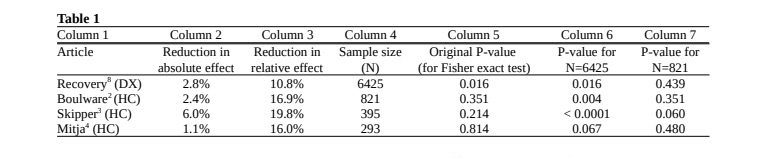
Columns 2 and 3 show the reduction in absolute and relative effect, respectively, for treatment groups in comparison to control groups. We display the effect for Recovery’s dexamethasone study on the percentage of deaths in hospitalized patients. For Boulware’s study the effect is shown in terms of the percentage of symptomatic outcomes in exposed participants.
For Skipper’s study we show the effect on the percentage of exposed participants with ongoing symptoms after 14 days.
For Mitja’s (4) study the effect is in terms of the percentage of hospitalized outcomes during a period of 28 days in patients with initially mild symptoms.
All four papers show mean improvements in their respective outcomes, but these variables are distinct from each other and thus columns 2 and 3 are not directly comparable. On the other hand, columns 6 and 7 are comparable.
Column 5 shows the original p-values of the studies for the respective sample sizes. Note that the only statistically significant result, at 95% level, is obtained for dexamethasone (line 1). However, note also that the sample size N=6425 in this study is considerably larger than sample sizes in all three hydroxychloroquine studies: 821, 423, 293.
To illustrate how much the sample sizes may influence the original p-values obtained, we calculate in columns 6 and 7 the hypothetical p-values we would have obtained for the same absolute and relative effects in each study, keeping the same proportions obtained in each study for both control and treatment groups, but equalizing the sample sizes to the same size of the two larger studies.
If all studies had sample size N=6425, column 6 shows that in the Boulware(2) and Skipper(3) papers the hydroxychloroquine treatment would possibly have a more significant p-value than the dexamethasone study, though we emphasize that these p-values are merely illustrative and cannot be considered as estimates.
Conversely, with sample sizes of 821, 395 and 293 patients the dexamethasone effect size would be non significant and have p-values equal to 0.439, 0.621 and 0.667 respectively. Its proportional p-value would be less than 0.05 only for a sample larger than 4228. In these cases, the p-values can be considered as formal estimates.
Hence, if the Recovery trial had the same sample size of the largest early treatment hydroxychloroquine trial there would be a high probability that the null hypothesis would have not been rejected and that dexamethasone would thus not be recommended to COVID-19 patients.
These last examples show how much the p-value can be affected by the sample size and that interpretations based only on p-values may lead to improper conclusions.
These comparisons bring some light to the discussion whether the lack of statistical significance in early treatment hydroxychloroquine trials were due to treatment effects or to small sample sizes. It becomes clear that it is not possible to affirm that early treatment of COVID-19 patients with hydroxychloroquine is not effective as the conclusions state.
On the contrary, the evidence from all these three randomized trials points to treatment effectiveness. If on one hand uncertainty may create false positive effects, on the other hand it may also mask positive effects even greater than the positive effects that have been measured so far.
Hence, we emphasize that larger studies are still necessary to decrease uncertainty and confirm these positive evidences.
Due to the importance of clinical trials in COVID-19 public decision making, we believe it is fundamental that these three studies correct their conclusions and publicize these corrections. In a pandemic the urgency of publication is justified and more errors might appear.
Nevertheless, best scientific practices, including proper data interpretation, must not be laid aside. As the American Statistical Association statement affirms “reduce data analysis or scientific inference to mechanical “bright-line” rules (such as “p < 0.05”) for justifying scientific claims or conclusions can lead to erroneous beliefs and poor decision making”.(9)
This open letter is signed by statisticians, medical researchers, clinicians and other quantitative researchers. The full list of signatories and affiliations can be found below.
Articles’ conclusions
Here we copy the conclusions of the three hydroxychloroquine articles discussed in the text above.
Boulware et al.(2)
https://www.nejm.org/doi/full/10.1056/NEJMoa2016638
Main conclusion (in abstract): “hydroxychloroquine did not prevent illness compatible with Covid-19 or
confirmed infection when used as postexposure prophylaxis within 4 days after exposure.”
Discussion: “In this trial, high doses of hydroxychloroquine did not prevent illness compatible with Covid-19
when initiated within 4 days after a high-risk or moderate-risk exposure”
Skipper et al.(3)
https://www.acpjournals.org/doi/full/10.7326/M20-4207
Main conclusion (in abstract): “Hydroxychloroquine did not substantially reduce symptom severity in
outpatients with early, mild COVID-19.”
“Overall, hydroxychloroquine failed to cause a statistically significant decrease in symptom prevalence or
severity over the 14-day study period.”
“This builds on other randomized trial data on hydroxychloroquine, which have not shown any benefit for
postexposure prophylaxis.”
Mitjà et al.(4)
https://academic.oup.com/cid/article/doi/10.1093/cid/ciaa1009/5872589
Main conclusion (in abstract): “In patients with mild Covid-19, no benefit was observed with HCQ beyond the
usual care.”
Discussion: “The results of this randomized controlled trial convincingly rule out any meaningful virological or
clinical benefit of HCQ in outpatients with mild Covid-19.”
References
- Horby et al., Effect of Hydroxychloroquine in Hospitalized Patients with COVID-19: Preliminary results from
a multi-centre, randomized, controlled trial. Doi: https://doi.org/10.1101/2020.07.15.20151852 - Boulware DR, Pullen MF, Bangdiwala AS, et al. A randomized trial of hydroxychloroquine as postexposure
prophylaxis for Covid-19. N Engl J Med (2020). Doi: 10.1056/NEJMoa2016638 - Skipper, C. et al., Hydroxychloroquine in Nonhospitalized Adults With Early COVID-19: A Randomized
Trial. Annals of Internal Medicine. https://doi.org/10.7326/M20-4207 - Mitjà, O. et al., Hydroxychloroquine for Early Treatment of Adults with Mild Covid-19: A Randomized-
Controlled Trial. Clinical Infectious Diseases, ciaa1009, https://doi.org/10.1093/cid/ciaa1009 - Makin, T. and Orban de Xivry, J. Science Forum: Ten common statistical mistakes to watch out for when
writing or reviewing a manuscript: Over-interpreting non-significant results. eLife 2019;8:e48175 DOI:
10.7554/eLife.48175 - Amrhein, V., Greenland, S. and McShane, B. Scientists rise up against statistical significance. Nature 567,
305-307 (2019). DOI: 10.1038/d41586-019-00857-9 - Editorial, Coronavirus drugs trials must get bigger and more collaborative. Nature 581, 120 (2020) Doi:
10.1038/d41586-020-01391-9 - The RECOVERY Collaborative Group, Dexamethasone in hospitalized patients with Covid-19 – Preliminary
Report. N Engl J Med (2020). DOI: 10.1056/NEJMoa2021436 - Ronald L. Wasserstein & Nicole A. Lazar (2016) The ASA Statement on p-Values: Context, Process, and
Purpose, The American Statistician, 70:2, 129-133, DOI: 10.1080/00031305.2016.1154108
Correspondence to letter.rct.statistics@gmail.com
To endorse the letter send an email with your name, degree and affiliation to letter.rct.statistics@gmail.com
List of Signatories
- Marcio Watanabe, PhD Statistics Universidade de São Paulo (Department of Statistics/Universidade Federal
Fluminense; Brazil) - Amber D. Bethea, PA-C MBA Health Care University of Miami (Department of Cardiology, Baylor Scott & White
Heart and Vascular Hospital; USA) - Bernardo Borba Andrade, PhD Statistics University of Minnesota (Department of Statistics/Universidade de
Brasília; Brazil) - Cláudia N. Paiva, PhD Biophysics Universidade Federal do Rio de Janeiro (Department of
Microbiology/Universidade Federal do Rio de Janeiro; Brazil) - Cristiana Altino de Almeida, MD Universidade Federal de Pernambuco (Former President of the Brazilian Society
of Nuclear Medicine; Brazil) - Daniel Victor Tausk, PhD Mathematics Universidade de São Paulo (Department of Mathematics/Universidade de
São Paulo; Brazil) - Dina Goldin, PhD Computer Science Brown University (School of Engineering/University of Connecticut; USA)
- Edmund Fordham, PhD Physics Cambridge University (independent Consultant in Physics and Energy
technologies, formerly Scientific Advisor to Schlumberger Ltd; United Kingdom) - Edson de Faria, PhD Mathematics CUNY (Full Professor of Mathematics, Universidade de São Paulo; Brazil)
- Eliana Benedictis, MD Universidade de São Paulo (former Pharmaceutical Industry Clinical Research Director;
Brazil) - Flavio Abdenur, PhD Mathematics IMPA (private sector; Brazil)
12.Francisco Cardoso, MD Universidade Federal do Rio de Janeiro (Infectologist at Hospital Emilio Ribas, São Paulo;
Brazil) - George von Borries, PhD Statistics Kansas State University (Department of Statistics, Universidade de Brasília;
Brazil) - Gustavo L Carvalho, MD MBA PhD Medicine Universidade Federal de Pernambuco (Associate Professor of
Surgery, Universidade de Pernambuco; Brazil)
15.John E. McKinnon, MD MSc (Co-Director of the Translational & Clinical Research Center, Clinical Associate
Professor, Division of Infectious Diseases, Wayne State University; USA)
16.José Guilherme de Lara Resende, PhD Economics University of Chicago (Department of Economics/Universidade
de Brasília; Brazil)
17.José Tavares-Neto MD PhD Clinical Medicine Universidade de São Paulo (Full Professor of Infectious
Diseases/Universidade Federal da Bahia; Brazil)
18.Juan M. Luco, PhD Biochemistry Universidad Nacional de San Luis (Department of Chemistry, Universidad
Nacional de San Luis; Argentina) - Leonardo Pezza, PhD Chemistry Unesp (Department of Biochemistry and Organic Chemistry/ Universidade
Estadual Paulista Júlio de Mesquita Filho; Brazil) - Lorenzo Ridolfi, PhD Computer Science PUC-Rio (partner Etho Solutions in Data Science; Brazil)
- Luiz Ayrton Santos Junior, MD, PhD, Universidade Federal de Pernambuco (President of Brazilian Society of
Bioethics PI. Coordinator of Postgraduate Course of Women Health, Federal University of Piaui; Brazil) - Marcos N. Eberlin, PhD Chemistry Universidade Estadual de Campinas (Department of Chemistry, Mackenzie
Presbyterian University; Brazil) - Marcus Sabry Azar Batista, MD PhD Internal Medicine Universidade Federal de São Paulo (Professor of
Medicine/Universidade Federal do Piauí; Brazil) - Marcus Zervos, MD (Division Head, Infectious Diseases, Professor of Medicine, and Assistant Dean of Global
Affairs, Wayne State University School of Medicine; USA) - Marina Bucar Barjud, MD PhD Internal Medicine University of Zaragoza (University of San Pablo CEU; Spain)
- Mostapha Benhenda, PhD Mathematics Université Paris 13 (Data scientist/Melwy and COVIND Covid-19 clinical
data consortium; Switzerland) - Nise H. Yamaguchi MD, Ph.D. Clinical Oncology and Tumor Immunology University of São Paulo (Hospital
Israelita Albert Einstein/ Instituto Avanços em Medicina/ Instituto Nise Yamaguchi) - Norman E Lepor, MD FACC FAHA FSCAI (Past President, California Chapter, American College of Cardiology;
Geffen School of Medicine, University of California Los Angeles; USA)
29.Paolo Zanotto, PhD Virology Oxford University (Department of Microbiology/Universidade de São Paulo; Brazil)
30.Pedro L. O. Volpe, PhD Chemistry Unicamp (Department of Physical Chemistry/Universidade
Estadual de Campinas; Brazil)
31.Peter A. McCullough, MD MPH University of Michigan, (Professor of Medicine/Texas A&M University and Vice
Chief of Medicine/Baylor Heart and Vascular Institute; USA) - Rodrigo De Losso, PhD University of Chicago (Full Professor of Economics/Universidade de São Paulo; Brazil)
- Rudnei Dias da Cunha, PhD Computer Science Kent University (Full Professor of the Institute of Mathematics and
Statistics/Universidade Federal do Rio Grande do Sul; Brazil)
34.Sabas Carlos Vieira, MD PhD Medicine Universidade Estadual de Campinas (Oncocenter; Brazil)
35.Sang C. Cha, MD PhD Medicine Universidade de São Paulo (former President of Brazilian Medical Ultrasound
Society; Brazil)
36.Simone Gold, MD Chicago Medical School (FABEM Fellow American Board of Emergency Medicine; USA)
37.Steven Hatfill MD MSc University of Capetown (Adjunct Assistant Professor of Clinical Research, George
Washington University; USA) - Vijay Gupta, MA Economics, Econometrics & Machine Learning Consultant (former World Bank, USAID, Tech
Mahindra, Blackstone Group Technologies, E&Y India, BearingPoint USA; India)
Melbourne healthcare workers recruited for hydroxychloroquine prophylactic trial